Divide and Conquer: Language Models can Plan and Self-Correct for Compositional Text-to-Image Generation
Abstract
Despite significant advancements in text-to-image models for generating high-quality images, these methods still struggle to ensure the controllability of text prompts over images in the context of complex text prompts, especially when it comes to retaining object attributes and relationships. In this paper, we propose CompAgent, a training-free approach for compositional text-to-image generation, with a large language model (LLM) agent as its core. The fundamental idea underlying CompAgent is premised on a divide-and-conquer methodology. Given a complex text prompt containing multiple concepts including objects, attributes, and relationships, the LLM agent initially decomposes it, which entails the extraction of individual objects, their associated attributes, and the prediction of a coherent scene layout. These individual objects can then be independently conquered. Subsequently, the agent performs reasoning by analyzing the text, plans and employs the tools to compose these isolated objects. The verification and human feedback mechanism is finally incorporated into our agent to further correct the potential attribute errors and refine the generated images. Guided by the LLM agent, we propose a tuning-free multi-concept customization model and a layout-to-image generation model as the tools for concept composition, and a local image editing method as the tool to interact with the agent for verification. The scene layout controls the image generation process among these tools to prevent confusion among multiple objects. Extensive experiments demonstrate the superiority of our approach for compositional text-to-image generation: CompAgent achieves more than 10\% improvement on T2I-CompBench, a comprehensive benchmark for open-world compositional T2I generation. The extension to various related tasks also illustrates the flexibility of our CompAgent for potential applications.
Video
Overview
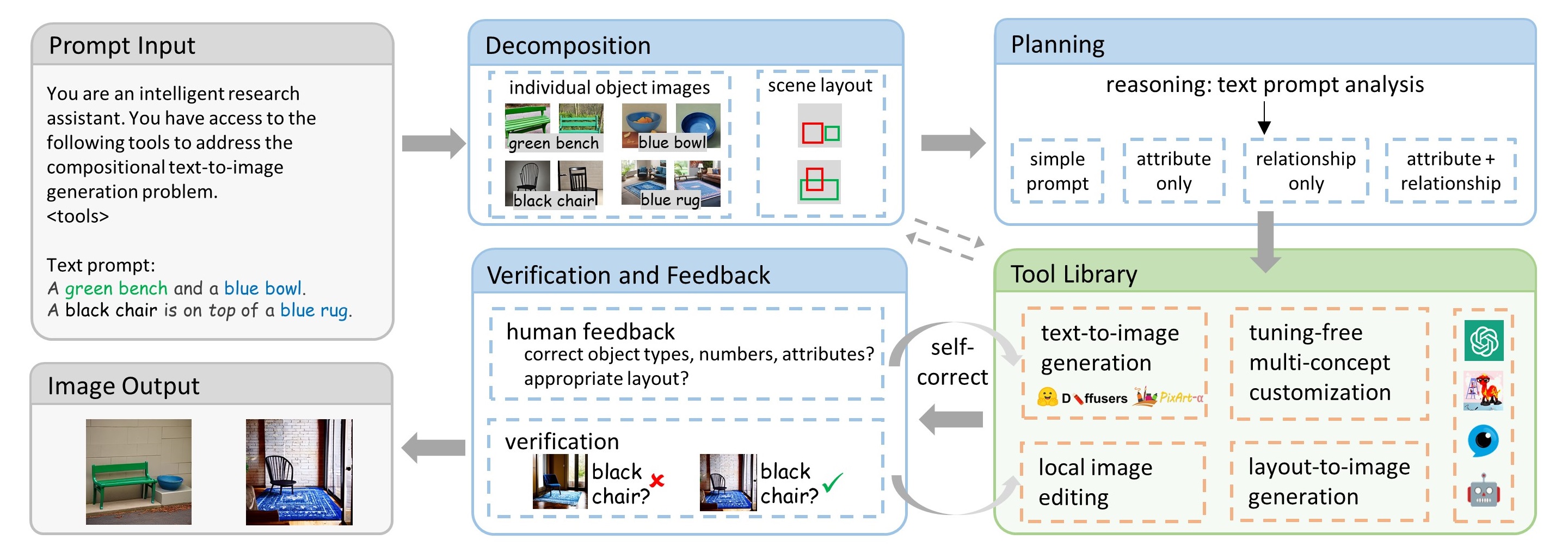
Overview of CompAgent: Given the input containing the complex text prompt, the LLM agent conducts the decomposition and planning tasks to invoke external tools for image generation. It then performs verification or involves human feedback and interacts with the tools for image self-correction. The final image output will well satisfy the requirements from the input text prompt.
Generation Results
CompAgent is able to generate accurate images given complex text prompts. For compositional-text-to-image generation, it well addresses the attribute binding and object relationship problems. Compared with existing methods, CompAgent generates images that better align with the description of complex text prompts.
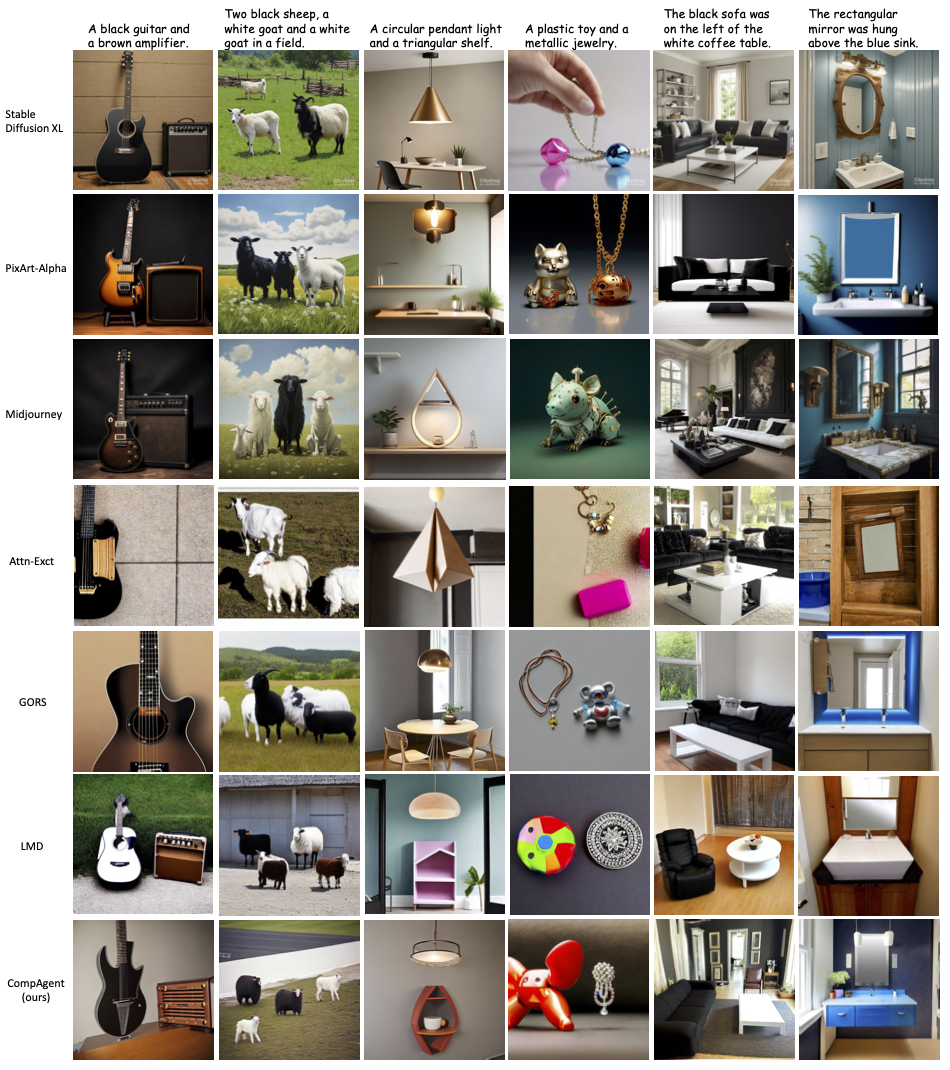
CompAgent generates accurately for attribute binding:
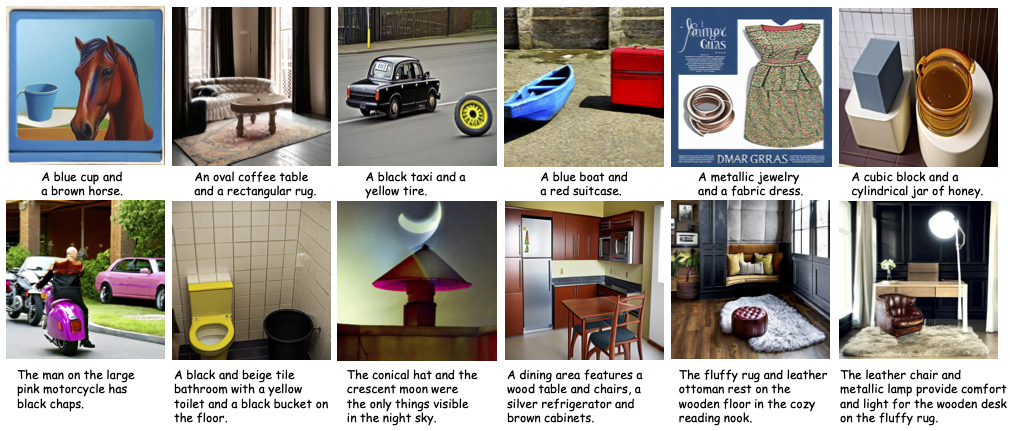
for object relationship:
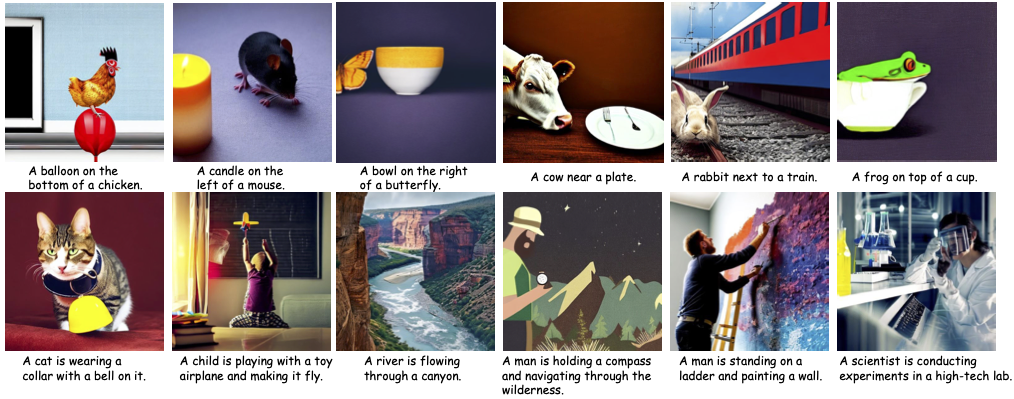
for complex compositions:
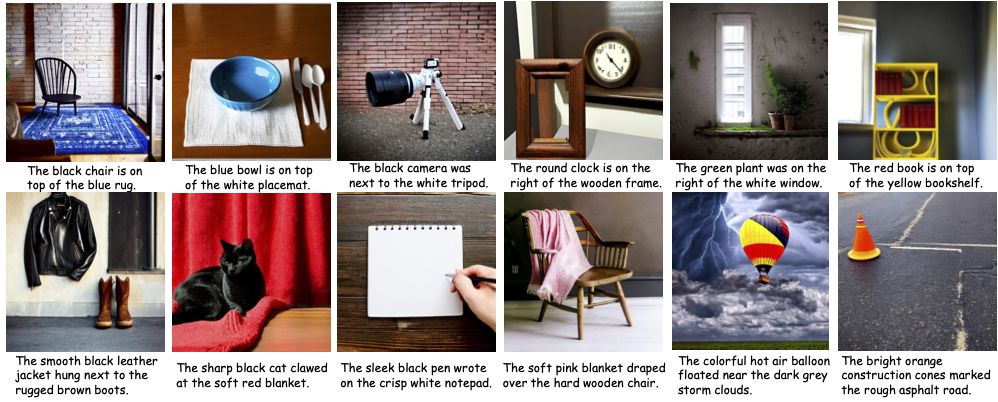
BibTeX
@article{wang2024div,
author = {Zhenyu, Wang and Enze, Xie and Aoxue, Li and Zhongdao, Wang and Xihui, Liu and Zhenguo, Li},
title = {Divide and Conquer: Language Models can Plan and Self-Correct for Compositional Text-to-Image Generation},
journal = {arXiv preprint arXiv:2401.15688},
year = {2024},
}